Systemization of Data Governance via a Data Governance Management System!
You’ve done Data Governance. But is it now time to systemize your Data Governance into an application?
In this article, we will outline a few reasons why realizing the value of data governance can be challenging and show how the systemization of data governance via a Data Governance Management System (DGMS) addresses these challenges. First, we’ll look at the definitions to understand the nuances of the terms. Next, we’ll look at several of the critical success factors required for successful Data Governance. Finally, we will discuss how the systemization of data governance is an accelerator for achieving embedded DG operations.
Let’s discuss a few definitions first for clarity and to lay out some of the nuances of the terminology used here. After all, we know that clarity of definition is the first principle of data governance! So, what is data governance?
Data Governance is:
The planning, oversight, and control of the organization’s data, and the implementation of the decision rights, roles and responsibilities, policies, processes, standards, and metrics to ensure that data is fit for use in meeting the business objectives. Each of these elements is created and organized in the following manner:
Based on the data management strategy and business objectives, the data governance authority develops the requisite executive charter, mission and scope, high-level accountabilities, and decision rights for data governance. An additional output is a policy that applies to data governance, such as valued data, verification and validation, and independent data stewardship. From the policies, the DG standards are derived, and from the standards, the DG metrics are derived.
To break this down a bit, the required parties, or people and role names — which are two sides of a coin — such as the DG Authority, with their business and technical colleagues, and the Data Stewards, oversee or conduct the mandatory DG processes of Compliance Review and Change Management.
The term “DG Authority,” which may refer to one person or many, is used to resolve the question of whether a steering committee, council, or other executive body is most senior when discussing multiple organizations. It represents the highest level of DG accountability that is relevant to the context in question. For example, at the policy level, the DG Authority is likely the team of Senior Business, IT, and DG leader(s) who are charged with DG policy development and approval. However, in the context of a workgroup conducting a change management process, the role name of DG Authority may be the Lead Data Steward. |
Technologies such as the data catalog or metadata repository, which provide significant value, would not be duplicated by a DGMS, but would receive guidance from it, as the DG system of record. Any DGMS should contain automated workflows which would be documented in the knowledge base.
Management process includes:
Planning, resourcing, organizing, directing/guiding, and controlling, and include communications planning and training. Sound management practices also include exception processing and conflict and issue resolution.
At their best, management processes also account for:
- Feedback loops for gathering input from those within its purview
- Documented procedures for vetting suggested improvements, and
- Implementation of managed changes as business requirements change.
When using the above practices, what is managed retains flexibility and adaptability while remaining within a disciplined framework. In this way, what is managed can be measured.
Data Governance Management
Data Governance Management means that specific guardrails are in place to ensure that the DG components of policies, roles, and standards are documented and aligned and that the DG processes occur in a predictable, reliable, repeatable manner to ensure consistency and reduce error and unwanted variance. Moving to managed data governance means that ad hoc DG activities are all but eliminated. At this increased level of maturity, DG Management encompasses the ability to bring any ad hoc activities into alignment, whether by training, conflict and issue resolution, exception management, or other means.
Resources such as centralized documentation serve as a rich knowledge base for training, communications, and standardization which contribute to the promulgation of data governance and its value.
A data governance management system (DGMS) standardizes and systemizes the practice of data governance management.
As an internally coherent system, a DGMS functions to:
- Provide all required elements for building data governance in the organization
- Track project or program progress when implementing data governance, and
- Measure the health of DG operations once it has been deployed.
The DGMS enables the early identification of success measures – derived from well-written policies and standards – that can then be replicated in other organizational areas. It can flag issues for early troubleshooting and resolution. It provides qualitative and quantitative metrics and insights into how the specified parts of the ongoing DG operations are working – and it is sufficiently flexible to easily tailor or customize to the organization’s needs. Further, it records and retains the metadata for governance activities which may be used for auditing purposes, as necessary. With all of this in place, the DGMS can become the DG System of Record.
It is well known that the implementation of DG is complex. It faces the many barriers of lack of executive-level support, DG policies, data literacy, training, or communication; confusion of responsibilities, roles, and processes; and inability to visualize or report results and success measures. Lacking a roadmap and plan, the misidentification of critical dependencies, and the sub-optimal sequencing of activities causes missteps along the way.
How a DGMS Benefits Your DG Initiatives
A critical success factor (CSF) is a key area where satisfactory performance is required for the organization to achieve its objectives. The value of a Data Governance Management System (DGMS) can be measured by the critical success factors of time savings, resource efficiencies, process performance, and value gained, which the system satisfies in qualitative or quantitative terms.
Naturally, there is an interconnectedness of CSFs that overlap across multiple or all four factors above. For example, the reduction or elimination of rework is a top driver for each of the four critical success factors, because if something must be done over again, it not only wastes time and resources but there is an opportunity for the introduction of further error and so further rework, compromising performance and value.
The application of a data governance management system for the implementation and deployment of Data Governance accelerates the achievement of the data governance critical success factors by providing the following:
- Prebuilt knowledge base with content and templates in the functional areas of data governance, including communications planning, training, and education
- Predefined data governance roles, responsibilities
- Predefined data governance workflows with metrics
- Roadmap, plan, and metrics for data governance deployment
Having a prebuilt data governance knowledge base prevents the uncertainty of where to start or borrowing from different popular sources that don’t fit together. The knowledge base supports the numerous staff resources and stakeholders who are involved in the DG activities. It provides expertise all in one source, with a common vocabulary and definitions, improving data literacy across the organization. It accelerates time to deployment, improves consistency, and decreases the chance of error. Prebuilt templates for the DG executive charter, policies, and processes and their metadata are included to provide time-savings, consistency, and the foundations of DG metrics.
The role names that conduct data governance, such as Data Architect, Business Analyst, and Data Steward, should be defined in the system and mapped to the data governance processes. Next, these role names should be mapped to the RACI designations (Responsible, Accountable, Consulted, and Informed). The team members can then be mapped to the DG role names. This increases the clarity of hand-offs between tasks, and provides clear communication and consistency, improving process performance, all of which drive resource efficiencies and speed time to value.
Workflows are commonly documented in “swim lane” diagrams, showing tasks, dependencies, and role names, which make workflow updates easy. Prebuilt automated workflows provide consistency and are enormous time-savers, especially if the organization does not use a data catalog tool. However, if there is such a tool in place, diagrams are valuable for troubleshooting workflows in the data catalog tool or may serve as documentation in the knowledge base and, therefore, become available to a wider audience.
A roadmap for DG deployment should include a project plan, built from the functions and processes defined in the knowledge base. These are used to guide and measure the progress of the project or program toward operationalizing data governance. The plan should be scalable, as this is better suited to a staged roll-out or federated governance model.
There are many views on how to visualize the deliverables of the data governance process in real-time. One view of the metrics is based on the measures derived from the policy and standards metadata. The second view, a progress view relates to the workflow project and process metrics that are derived in the system from the use of the automated workflows of compliance review and change management. They describe variances, completion rates, and quality.
A great challenge of data governance is deciphering the many layers of the discipline, the interrelationships of the many components and processes, and deciding what to do next. The levels of conceptual abstraction are numerous, such as the uses of metadata. Organizations looking to reduce the heavy lifting of implementing data governance may want to systemize data governance.
The value of a DGMS is accelerated from time to value. The benefits of prebuilt content and organization of all the moving parts, discipline, communication, and reliable processes brought about by a DGMS are enormous and contribute to the promulgation of data governance and its value.
Given the accelerator capabilities of a Data Governance Management System with prebuilt content and processes, a general estimation suggests that an organization could save hundreds of hours and resources, compress the time-to-solution by many months, achieve time-to-value in a fraction of the cost of developing Data Governance traditionally.
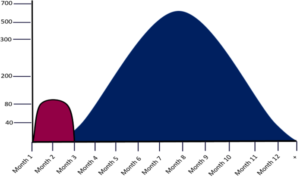
For more information on Sandhills approach to delivering Data Governance Management Systems (COMPASS), please contact Robert.Lutton@sandhillconsultants.com.